Welcome to BARS#
BARS is a project aimed for open BenchmArking for Recommender Systems https://openbenchmark.github.io/BARS
Despite the significant progress made in both research and practice of recommender systems over the past two decades, there is a lack of a widely-recognized benchmark in this field. This not only increases the difficulty in reproducing existing studies, but also incurs inconsistent experimental results among them, which largely limit the practical value and potential impact of research in this field.
Openness is key to fostering progress in scientific research. BARS is such a project aimed for open BenchmArking for Recommender Systems, allowing better reproducibility and replicability of quantitative research results. The ultimate goal of BARS is to drive more reproducible research in the development of recommender systems.
Key Features#
In summary, BARS is built with the following key features:
Open datasets: BARS collects a set of widely-used public datasets for recommendation research, and assign unique dataset IDs to track different data splits of each dataset. This allows researchers to experiment with the datasets and share the results in a consistent way.
Open source: BARS actively supports the open source principles and provides a list of open-source libraries of popular recommendation models.
Standardized benchmarking pipeline: BARS standardizes the open benchmarking pipeline for reproducible recommender systems research to ensure transparency and availability of all the artifacts produced along the process.
Benchmark leaderboard: BARS serves a leaderboard with the most comprehensive benchmarking results to date, covering tens of SOTA models and over ten dataset splits. These results could be easily reused for future research.
Reproducing steps: The core of BARS is to ensure reproducibility of each benchmarking result through detailed description of reproducing steps, which allows anyone in the community to easily reproduce the results.
Strong maintainability: BARS is open to the community. Anyone can contribute new datasets, implement new models, update new benchmarking results, or revise existing numbers through a pull request on Github. The contributor will be marked honorably along with the contributions accordingly.
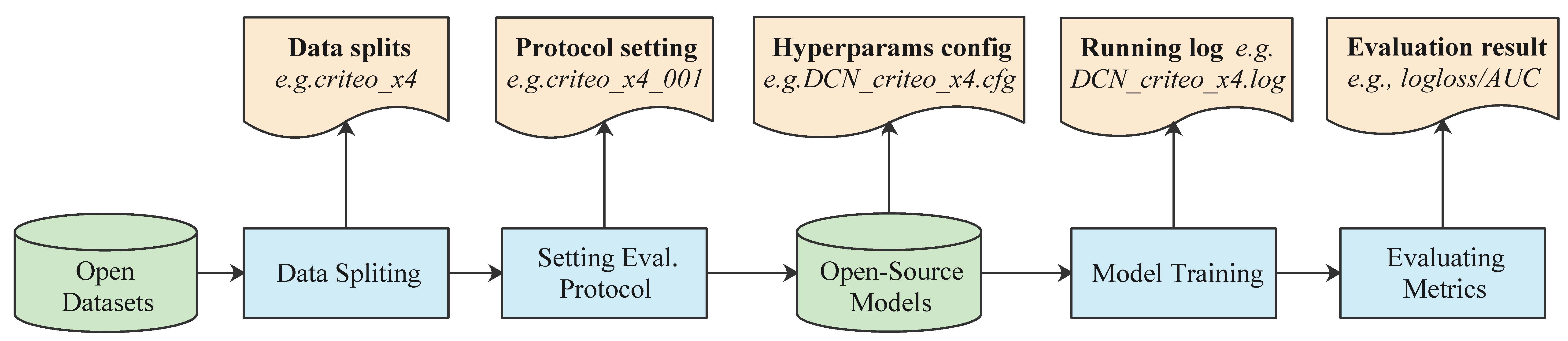
Fig. 1 The Open Benchmarking Pipeline#
By establishing an open benchmarking standard, together with the freely available datasets, source code, and reproducing steps, we hope that the BARS project could benefit all researchers, practitioners, and educators in the community.
Benchmark Tasks#
The BARS benchmark currently covers the following two main recommendation tasks.
Ongoing projects:
BARS-Rerank: An Open Benchmark for Listwise Re-ranking
BARS-MTL: An Open Benchmark for Multi-Task Ranking
Citation#
If you find our benchmarks helpful in your research, please kindly cite the following papers.
Jieming Zhu, Quanyu Dai, Liangcai Su, Rong Ma, Jinyang Liu, Guohao Cai, Xi Xiao, Rui Zhang. BARS: Towards Open Benchmarking for Recommender Systems. The 45th International ACM SIGIR Conference on Research and Development in Information Retrieval (SIGIR), 2022. [Bibtex]
Discussion#
If you have any questions or feedback about the BARS benchamrk, please start a discussion here, or join our WeChat group.